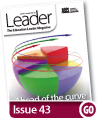
Data analysis
There is a mass of education data now available to schools and colleges but Mike Treadaway of the Fisher Family Trust fears that misuse of data is still a common problem. He offers some guidelines to help school and college leaders use data intelligently.
The last ten years have seen an enormous increase in the availability and use of education data in England and Wales. Since the early 1990s, the collection of test and teacher assessment data and the linking of this data with school census information has led to analyses being developed which support detailed self-evaluation and target-setting.
As long ago as 2000, when the Fischer Family Trust (FFT) started its Data Analysis project, a number of principles were felt to be important if data was to be used intelligently. These were included in training materials and guidance:
-
Data analysis should promote discussion, evaluation and planning. Analyses are provided to support internal self-evaluation. They are most effective when used in conjunction with local knowledge and experience.
-
Estimates are not targets. Estimates can be used to support discussion leading to the setting of appropriately realistic and challenging targets. Considering a range of estimates is more likely to promote effective discussion about expectations.
-
Data raises questions; it does not provide answers. Investigation and discussion lead to actions. Analyses support self-evaluation by highlighting areas where pupil progress is significantly different from that of similar pupils nationally. A combination of data analysis and professional judgement is more effective than either used alone.
When I revisited these early support materials I had two reactions. Firstly, the principles are just as important now, if not more so. Secondly, we still have a very long way to go to achieve the widespread implementation of these principles. Why is this so?
Let's contrast some examples of good use of data with others where these principles were certainly not followed. First, here are two ways to use data effectively:
A headteacher was concerned about attainment in maths at GCSE, particularly for a group of 'middle ability' boys. Working with a local authority adviser they looked at value-added for these students and noticed that it was at Key Stage 3 where their progress was well below average - and that the students had all been in the same teaching group. This led to actions to work with specific students in years 10 and 11 and support for the professional development of the teacher concerned.
As part of the process of thinking about targets and expectations, students were provided with information about the 'chances' of achieving different grades in each subject, based upon their previous attainment at Key Stages 2 and 3. They had opportunities to share this information with each other in small peer groups, leading to the development of realistic and ambitious targets - and to actions, strategies and support they would need to achieve these. Now compare that approach with these two examples:
Example 1: A student was given a 'target' (a single grade) for science at Key Stage 4 at the start of year 9. Because the school had not updated systems with most recent estimates the information used was based upon attainment at Key Stage 2. The student had made well above average progress during Key Stage 3, so the 'target' was well below what the student expected to get at GCSE. When the student and parents queried this they were told by the teacher that the data was accurate and that they were "not allowed to change the targets".
Example 2: A secondary school with contextual value-added shown as 'significantly lower than expected' for the previous three years was deemed to be failing. What had been ignored was that the school had been improving. Its rank (against all other schools nationally) had gone up from 99 to 90 to 80 over the three-year period. This meant that, whilst it still had some way to go, it was, in value-added terms at least, one of the fastest improving schools nationally.
While examples of the apparent misuse of data may be in the minority, they are sufficiently common to be a concern. So, what can be done to improve the situation? I would suggest that we need to take action on at least two fronts:
-
develop a code of practice and some key principles for the use of educational data which, if followed, would be likely to ensure that data was used effectively and with appropriate sensitivity
-
improve our understanding of the impact on users of exactly how data is presented in reports and use this knowledge to improve systems at all levels - national, management information systems and bespoke systems developed within schools
What key aspects of data literacy might such a code of practice include? Here are some thoughts, with examples to illustrate how they might be applied.
Triangulation
Ideally, two or more sources of data should be compared and evaluated along with other evidence. If all give a similar message, that should be a solid basis for action. Where they say very different things then the reaction should be to investigate further.
A student might, for example, have an assessment based upon a test early in year 7 which shows much lower attainment than s/he achieved at the end of Key Stage 2.
It could be that the previous assessment was inflflated but it is just as likely that the pupil has not settled in well in the first couple of weeks in the new school. Just because two items of data say different things it does not mean that one must be wrong - they could both be wrong!
Statistical significance
The use of statistical significance on reports and analyses can help to distinguish between cases where differences appear large but are based only on a small number of cases. Value-added analysis should always show whether or not a score is classed as statistically significant.
Trends
Looking at trends, over at least three years, is a vital part of making a balanced assessment. It becomes even more important when looking at groups of pupils or individual subjects within schools, particularly where numbers are small. Trends can also be important in identifying improvement - especially in cases where statistical significance does not change from year to year.
The use of statistical significance is also important when looking at trends. In the 'middle range' (where value-added scores are not significant) changes in percentile rank are likely to be meaningless - ranks of 50, 40 and 60 over three years simply mean that the score has been about average every year. However, the difference between, for example, the 20th and 10th percentile is much larger and likely to be significant.
So, when looking at trends, analyses should test whether the difference between two scores is significant.
Estimates, predictions and targets
A calculation based on applying some sort of statistical model to prior-attainment data provides an estimate. This shows, in effect, what an individual student might achieve if s/ he makes average progress. Estimates should never be used as targets without some form of moderation.
Predictions derive from estimates - they take into account other information. A teacher might, taking an estimate as a starting point, feel that the likely attainment is going to be well above, similar to or well below the estimate. Predictions are, therefore, what you think will happen if nothing additional is done.
Targets are about deciding how much improvement to aim for. This will involve taking account of a range of other information, including levels of ambition, resources, and available support strategies.
Chances
Estimates should emphasise the use of chance, or how likely something is to happen. Considering a range of possibilities - whether looking at individual students or at summaries for a group of students - is likely to generate thinking along the lines of: "If 20 per cent of students like me achieved this last year then maybe I can. What do I need to do to achieve this?"
Chances also work well when they are averaged to give an overall estimate for a group. Adding up estimates which provide only a 'most likely grade' will almost always give an incorrect estimate for the group.
From principles to practice
I won't pretend that achieving this is easy. Even where the issues are understood there are pressures of time and limitations of existing systems to consider. A recent discussion with some schools about the use of chances data for Key Stage 4 subject estimates led to feedback along the lines of: "Well, we know that using chances data is educationally better but our management information system can only accept a single grade."
The recent report from the task group on Assessment and Testing included a recommendation that work should be done to "establish a protocol illustrating how data can be used and misused and inviting schools to commit themselves to a good practice guide."
The last ten years have seen an enormous growth in the amount of data available. What is needed now is a similar growth in the level of data literacy among all who use educational data to inform decisions.
More than 90 per cent of secondary schools now use Fischer Family Trust (FFT) Live - the primary source of FFT data for schools and local authority officers - on a regular basis. As part of training and support for analyses provided by FFT we are hoping to be able to take data literacy forward over the coming year. If you are interested in contributing to this work please email support@fischertrust.org
Mike Treadaway is director of research at Fischer Family Trust.
CVA and other data issues
ASCL has regular meetings to consider data issues with both the DCSF and Ofsted. These meetings are very constructive and we are able to raise matters of both policy and technical detail. Current issues under discussion include:
-
The impact of the annual change in coefficients on CVA values
-
The change from CVA to CVA plus English and maths and the impact on values
-
Impact of curriculum provision on CVA scores
-
How the baselining of CVA proposed in the school report card prospectus will work in practice
-
Splitting the data on levels of progress indicator by input level
-
The use of data by Ofsted and the impact on inspection judgements
-
Impact of level 3 volume on the post-16 CVA
-
The potential issues emerging from E grades virtually always giving negative value added in the post-16 CVA
-
Investigating why many high achieving schools on raw scores post-16 have relatively low value added (in many cases this is a general studies effect)
-
The potential impact of treating all subject grading equally with no allowance for some subjects being 'more severely' graded (as indicated in the University of Durham research
Members who have specific queries or issues with the workings or use of CVA should email ASCL policy assistant, Joe Liardet at joe.liardet@ascl.org.uk
© 2025 Association of School and College Leaders | Designed with IMPACT